Harnessing Medical Datasets for Machine Learning: A Comprehensive Guide
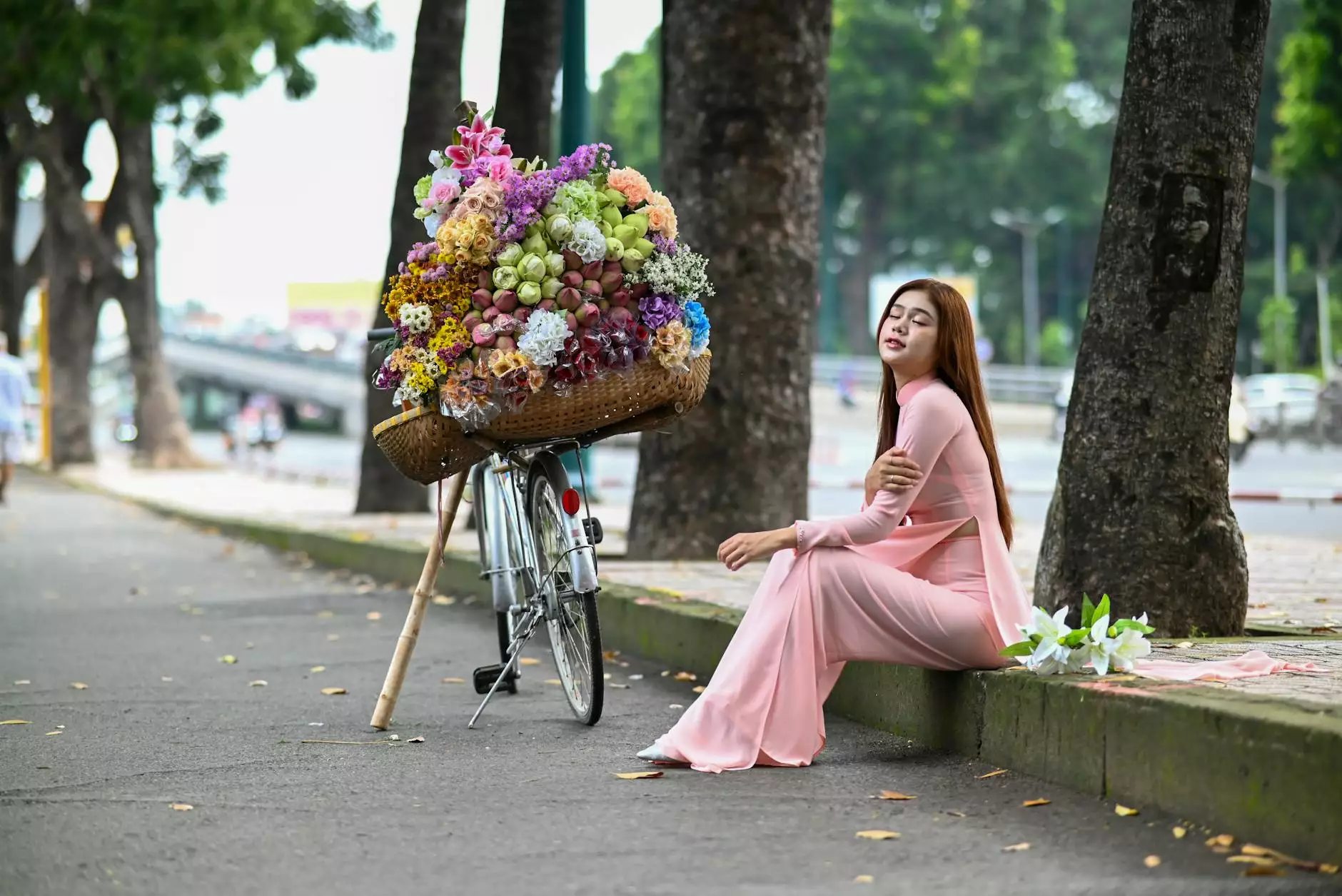
In the digital age, the integration of technology into everyday life has revolutionized various sectors, with the healthcare industry standing out. Machine learning has emerged as a transformative tool, and medical datasets for machine learning are at the forefront of this shift. This article explores how these datasets are constructed, their significance, and their direct implications on home services, especially in the realm of keys and locksmiths, as facilitated by businesses like KeyMakr.
Understanding Medical Datasets
At the core of every machine learning application lies the dataset. A medical dataset consists of various types of data collected from patients, hospitals, and research studies. This data can include:
- Clinical data: Information derived from patient treatments, diagnoses, and outcomes.
- Demographic information: Patient characteristics including age, gender, race, and location.
- Medical imaging: Radiological images used for diagnosing illnesses.
- Genetic data: Information pertaining to genetic predispositions to diseases.
With the rise of big data, the volume and variety of medical datasets have expanded, allowing for deeper insights and more accurate predictions in the healthcare field.
The Role of Machine Learning in Healthcare
Machine learning plays a crucial role in analyzing medical datasets. By leveraging algorithms that recognize patterns within data, healthcare professionals can make informed decisions regarding patient care. Some of the ways machine learning is utilized in healthcare include:
- Predictive Analytics: Using historical data to predict future outcomes such as disease progression.
- Personalized Medicine: Tailoring treatment plans based on the unique genetic makeup of individuals.
- Diagnostic Tools: Enhancing the accuracy of diagnoses through data-driven analysis.
- Operational Efficiency: Streamlining hospital operations and improving resource allocation.
Building Effective Medical Datasets for Machine Learning
Creating an effective medical dataset for machine learning involves several steps:
1. Data Collection
The foundation of any dataset is robust data collection. This can involve:
- Conducting surveys and questionnaires.
- Gathering electronic health records (EHRs) from healthcare institutions.
- Utilizing wearable technology that tracks patient health metrics.
2. Data Integration
Once the data is collected, it often comes from disparate sources. Integration involves consolidating data into a unified format. This step ensures compatibility with machine learning algorithms.
3. Data Cleaning
Quality control is essential. Data cleaning involves:
- Removing duplicate entries.
- Correcting inaccuracies and inconsistencies.
- Handling missing data through imputation or removal.
4. Data Labeling
For supervised learning models, data needs to be labeled accurately. This allows the model to learn from known outcomes, enhancing its accuracy when making predictions.
5. Continuous Updating
Medical datasets should evolve over time. Regular updates ensure that the data reflects the current reality of medical practices and patient populations.
Ethical Considerations in Medical Data Usage
Using medical datasets for machine learning raises various ethical questions including:
- Patient Privacy: Ensuring data is anonymized to protect patient identities.
- Bias in Data: Recognizing and mitigating bias that may lead to unequal healthcare outcomes.
- Consent: Obtaining informed consent from patients before collecting and utilizing their data.
Applications of Medical Datasets in Home Services
The application of machine learning through medical datasets extends to home services, especially for businesses like KeyMakr that offer locksmith and key services. Here are some insights into how this technology can improve service efficiency:
1. Emergency Response Optimization
Machine learning algorithms analyzing health-related datasets can predict which areas may experience higher emergency call rates. Consequently, locksmiths can be proactive in positioning their services efficiently during peak times, ensuring assistance is readily available when needed.
2. Enhancing Customer Service
Data analytics can help KeyMakr understand customer needs and preferences. By evaluating past service requests, they can streamline operations and anticipate customer demands.
3. Fraud Prevention
By applying predictive analytics from medical datasets, businesses can implement enhanced security measures to detect potential fraud in service requests. This ensures the safety of both customers and service providers.
The Future of Medical Datasets and Machine Learning
The future of medical datasets for machine learning holds immense promise. With the continued advancement of technology, we can expect the following trends:
1. Increased Accessibility
As regulations evolve, access to high-quality datasets is becoming more widespread, allowing a broader range of researchers and developers to innovate within the healthcare space.
2. Enhanced Artificial Intelligence
Deep learning technologies will become more sophisticated, providing even clearer insights from medical datasets, and improving predictions across multiple dimensions of healthcare.
3. Global Collaboration
International collaborations will lead to the creation of more comprehensive datasets that include diverse populations, further enhancing the accuracy of machine learning models and contributing to a more equitable healthcare system.
Conclusion
Utilizing medical datasets for machine learning is a game-changer in the healthcare sector, fueling advancements that not only enhance patient outcomes but also improve overall service efficiency in related fields, including home services provided by companies like KeyMakr. By focusing on data collection, processing, ethical considerations, and future applications, we can harness the full potential of this revolutionary technology. As we move forward, the interplay between healthcare and technology will continue to unfold, promising a healthier future driven by data-informed decision-making.